Solution Provider Maturity Index (SPMIND)
SPMIND allows a solution provider, developing or integrating solutions based on Artificial Intelligence (AI), to be recognized as a trusted provider for building and deploying such AI solutions.
Not Qualified – Progress to be Made | Qualified – Exploratory Level | Qualified – Intermediate Level | Qualified – Advanced Level | |
Average Score | Less than 2.5 | 2.5 to 3.4 | 3.5 to 4.5 | More than 4.5 |
General Capabilities | Mastery of AI issues is not sufficient | Mastery of AI issues is sufficient, with some dimensions not fully covered | Mastery of AI issues is sufficient, most dimensions are fully covered | Mastery of AI issues is sufficient, with all dimensions well covered |
General Characteristics | May not fully identify pitfalls of AI project | Masters most aspects of AI projects, but may fail in some relatively easy cases | Masters most aspects of AI projects, but may fail in hard cases | Masters all aspects of AI projects, can be expected to succeed in all cases |
Solution Provider Qualification | Cannot be a trusted provider for SMEs | Can be a trusted provider for SMEs on simple cases | Can be a trusted provider for SMEs on hard cases in some domains | Can be a trusted provider for SMEs in all cases |
SPMIND Components
SPMIND consists of 6 pillars with each pillar having requirements to assess whether the solution provider has achieved a satisfying level of AI maturity.
Organizational maturity and corporate governance evaluate whether the organization has put in place an organizational and governance system enabling the success of AI projects. Commercial maturity examines the organization’s ability relating to commercialization and marketing of their AI solution, its skills not being limited only to the design of the system. Data maturity checks whether the organization understands and controls the data and is aware of the associated obligations and issues. Ethics maturity evaluates whether the organization has sufficient understanding and control to deploy impact-controlled AI. Infrastructure maturity validates that the organization can rely on a hardware and software infrastructure to ensure the technological maturity and deployment of the solution. Finally, the end-to-end maturity checks whether the organization demonstrates knowledge and mastery of all system engineering phases, and appropriate consideration of the target operational context.
Collectively, the 6 pillars of SPMIND provide a complete assessment of an organization’s qualification for becoming a trusted AI Service Provider.
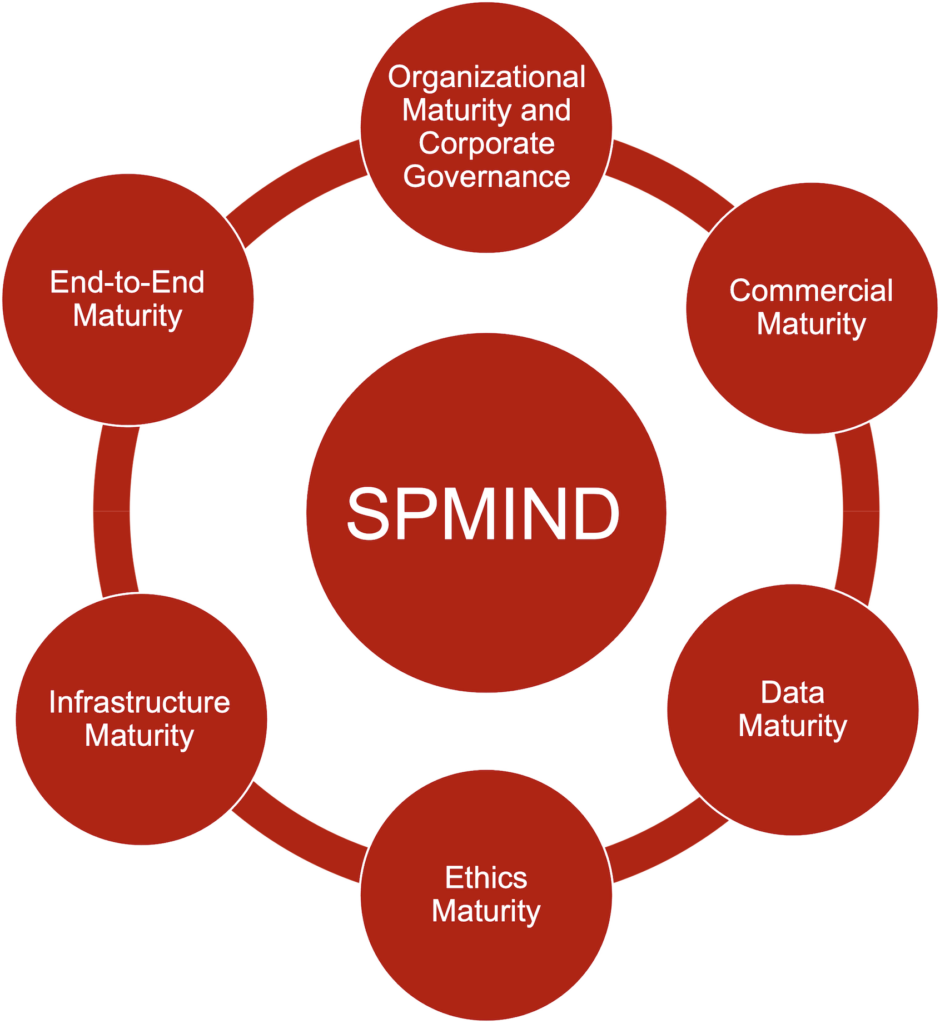
The 6 pillars and 36 dimensions assess a specific area that contributes to the overall solution provider maturity.
In each topic, it has several dimensions and each dimension is assessed at four levels of solution provider maturity:
- Not Qualified – Progress to be made
- Qualified – Exploratory Level
- Qualified – Intermediate Level
- Qualified – Advanced Level
Scores on each dimension are averaged to obtain a score at the pillar level.
Pillars | Dimensions | Assessment |
Organizational Maturity and Corporate Governance | Understanding of the value chain | The organization can identify the stages in the value chain into which his offer will fit. The extent of the value chain and the nature of its stages will depend on the specific context of the applicant (B2B or B2C, etc.). The value chain must cover a minima possible end users, purchasers of the solution and subcontractors. |
Decision-makers’ AI culture | The direction and management of the company concerned by the AI projects have an AI culture and sufficient understanding of the needs, constraints, limitations and risks associated with AI projects. | |
AI skills of staff involved in AI projects | The personnel involved in all the engineering phases necessary for the development and deployment of AI (including the software and data aspects) are identified and the company implements means to ensure their skills. | |
Control of dependencies and subcontracting | The organization analyzes the criticality of digital dependencies (software, libraries, data) and/or relationships with subcontractors, with regard to the AI project (total, partial or minority dependencies) and provides remedial strategies in the event of breakdowns in these dependencies and/or relationships. | |
Commercial Maturity | Mastery of the business use case | The organization is able to clearly present the different uses (sector of activity, specific technological expectations, customer size, etc.) for which he intends his AI solutions. Uses may concern both external customers and interlocutors within the company. |
Consistency of business strategies | The organization implements a business strategy consistent with the nature of the proposed AI solution. If the “AI” argument is not highlighted in its marketing strategy, explain the relevance of this choice. | |
Relevance of development and deployment strategies | The organization implements development and deployment strategies adapted to the uses for which he intends his products/services (type of customer -SME, ETI, etc.- the criticality of the sector, the structure of the customer’s company, or the customer’s development and deployment standards | |
Synergies with the ecosystem | The organization maintains links with the relevant ecosystem (incubators, competitiveness clusters, participation in projects, scientific contributions, etc.). | |
Administrative and tax compliance | The organization must be registered in the trade and companies register and have paid the taxes and social security contributions due on the date of the application. | |
Go-to-market experience | The organization carries out actions relating to the placing on the market of the AI solution, e.g. actions carried out with customers, actions relating to regulatory compliance related to the final application domain of the AI solution, actions of the sales department, etc. | |
Customer References | The organization is able to present customer references: attestation of success of a sales action or integration of an AI solution with a customer or references from proofs of concept (POC) if the organization has not yet carried out a sales action. | Data Maturity | Data governance | The organization implements a process allowing to clearly identify the different data sets used as input and generated as output, to know the origin of the data and whether they are managed internally or externally, and to identify the persons responsible for the processing carried out on this data. |
Mastery of the data lifecycle | The organization is able to explain, for each stage of the data life cycle, the methods used for identifying the needs and constraints associated with each stage. These stages are expected to cover all the processing and operations carried out on the data from its creation until its deletion. | |
Fairness and legality of data processing | The organization implements methods allowing the identification of data and metadata which may potentially cause unfairness in the processing carried out by AI and of elements whose processing may not comply with regulation depending on the application cases. | |
Mastery of access rights | The organization implements methods to restrict the model’s access to data likely to cause unfairness or illegal processing, both during the development phase and in operational conditions. | |
Mastery of quality | The organization implements data quality monitoring processes throughout the data life cycle. The organization proposes data quality attributes that are relevant to his context. | |
Mastery of data corpus preparation | Within the framework of learning the models, the organization implements methods for splitting the data sets in accordance with best practices in the field (only applicable to certain types of AI solutions). | |
Mastery of data under operational conditions | The organization implements methods to ensure that the input data under operational conditions is similar to the data used to create the models (only applicable to certain types of AI solutions). | Ethics Maturity | Ethics Awareness | The organization carries out actions relevant for raising awareness of ethics among all personnel involved in AI projects. |
AI-specific risk management | The organization implements a risk-based approach, which covers in particular the risks specific to the use of AI, and also includes the risks for fundamental rights (society, individual impact). (Only organization’s ability to identify risks is assessed not the quality and appropriateness of risk reduction strategies ). | |
Fairness of the processing carried out under operational conditions | The organization implements a risk-based approach, identifying the risks of unfairness in operational conditions. (Only organization’s ability to identify risks is assessed not the quality and appropriateness of risk reduction strategies). | |
Explainability and interpretability | The organization implements methods to ensure that end users and people impacted by the processing carried out by the AI solutions are informed of the elements that led to the production of the system’s outputs. The information received is suitable for the use of these people. | |
Traceability of decision-making | The organization implements methods to trace the data that led to an output from the AI solution. The organization must at least show that it implements strategies to keep a history of the processing carried out. | |
Transparency | The organization provides technical documentation specifying in particular the types of algorithm implemented, the expected performances and the field of use (internal use or for certain interlocutors of the organization). | |
Stakeholder information | The organization implements strategies allowing the stakeholders (development, use, inspection, general public, etc.) to have the information elements necessary for their relationship with the AI solution. | |
Environmental impact | The organization implements strategies to limit the impact of its solution on the environment. These strategies can relate to the data, the algorithm, or internal processes. | |
Infrastructure Maturity | Control of the data infrastructure | The organization formalizes the specifications of the infrastructure necessary for each stage of the data life cycle and makes sure to implement the resources (material and human) necessary for the proper functioning of the infrastructure at each stage (these stages cover all the processing and operations carried out on the data from its creation until its deletion). |
Mastery of system engineering infrastructure | The organization formalizes the specifications of the infrastructure required at each stage of the system engineering cycle and makes sure to implement the resources (material and human) necessary for the proper functioning of the infrastructure at each stage (these stages cover at least specification, development, verification and validation, deployment and maintenance). | |
Control of the infrastructure in operational conditions | The organization formalizes the specifications of the necessary infrastructure in operational conditions, and either adapts his system to the customer’s infrastructure, or informs his customer in an appropriate manner of the resources (material and human) necessary for the smooth operation of the system. | |
Protection and security | The organization implements data protection strategies at each stage of the system engineering cycle, e.g., GDPR policy. | |
End-to-End Maturity | Control of the design | The organization formalizes the basic principles of its AI solutions by taking into account the needs relating to the target operational context (type of users, business constraints, etc.) and technical constraints. |
Proficiency in data engineering | The organization formalizes the necessary operations within the framework of the data engineering stages, and sets up appropriate resources (software, hardware and personnel). | |
Mastery of model development | The organization formalizes the necessary operations as part of the selection and development of the AI model, and sets up appropriate resources (software, hardware and personnel). | |
Mastery of system implementation | The organization formalizes the operations necessary for the implementation of the complete system, and sets up appropriate resources (software, hardware and personnel). | |
Proficiency in verification | The organization implements a structured approach for carrying out verifications of AI solutions. Verification methods go beyond unit tests for proper functioning, cover complete AI solutions and not just models, and the performance indicators used are adapted to the types of performance sought by the client. | |
Control of deployment | The organization implements procedures to ensure proper use of the system, monitoring of the system once deployed, and allowing maintenance operations to be carried out. |